Business Analytics: Importance of Real-Time Data Analytics in Financial Decision-Making
In today’s fast-paced financial landscape, real-time data analytics has emerged as a game-changer. Businesses and financial institutions are leveraging data analytics to make informed decisions, reduce risks, and maximize profits.
With the increasing availability of big data and advanced analytical tools, real-time insights are revolutionizing financial decision-making. The ability to process data in real-time enables swift responses to market changes.
Companies can analyze and act on data quickly. This allows them to mitigate potential threats and seize new opportunities.
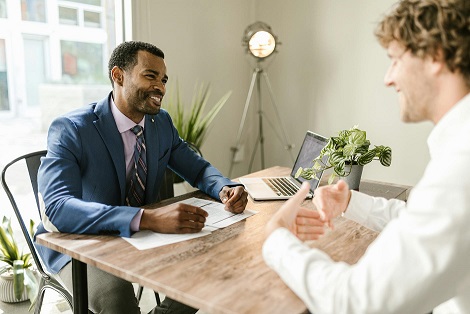
Real-time analytics is further underscored by the rapid digital transformation occurring across industries. Traditional financial decision-making relied heavily on historical data, often leading to delayed responses to market fluctuations.
Today, with real-time analytics, companies can monitor transactions, detect anomalies, and improve investments as events unfold. This shift toward real-time processing is crucial in maintaining a competitive edge in an increasingly data-driven world.
Financial institutions, investment firms, and enterprises that embrace real-time data analytics are positioned to enhance their agility and efficiency.
Whether it’s detecting fraudulent activities, refining risk management strategies, or improving customer experiences, real-time business analytics enables smarter, data-driven decision-making. Let’s explore the role of business analytics in finance.
Before we dive into to the topic, first we need to understand. Leveraging real-time insights is not just a trend. It is a necessity in modern finance.
The Role of Business Analytics in Finance
Business analytics involves the use of statistical analysis, predictive modeling, and machine learning to interpret data and drive decision-making. Financial professionals can extract valuable insights from vast amounts of structured and unstructured data.
This enables proactive and strategic planning. By leveraging advanced analytics, organizations can assess past performance. They can also predict future trends and behaviors. These capabilities guarantee they stay ahead in an ever-evolving financial environment. In the financial sector, business analytics plays a crucial role in several key areas:
Risk Management
Risk management is one of the most critical applications of business analytics in finance. By utilizing data-driven insights, companies can recognize potential risks early and implement strategies to mitigate them before they escalate.
Predictive business analytics models evaluate historical data. They consider market conditions and examine external factors. These models anticipate potential downturns, credit risks, and operational vulnerabilities.
Financial institutions rely on risk assessment models to prevent losses, manage credit risk, and maintain regulatory compliance. Real-time monitoring of financial transactions also helps organizations respond quickly to potential threats and economic fluctuations.
Fraud Detection
With the increasing digitization of financial transactions, fraud detection has become a top priority for financial institutions. Business analytics enables organizations to spot anomalies and suspicious activities in real-time, reducing the risk of fraudulent transactions.
Machine learning algorithms analyze customer behavior patterns. They also review transaction histories. These processes identify irregular activities like unauthorized transactions, identity theft, and cyberattacks.
Real-time business analytics enhances fraud prevention mechanisms. It provides instant alerts and automated responses. These measures guarantee customer assets and sensitive data remain protected.
Investment Strategies
Investors and asset managers use business analytics to optimize portfolio management and enhance stock market predictions. Predictive modeling and AI-powered analytics help financial professionals. They analyze market trends. They assess economic indicators and company performance. This leads to informed investment decisions.
By leveraging big data, financial institutions can gain insights into emerging market opportunities. They can assess potential risks. They can also diversify investment portfolios suitably. Advanced analytics power algorithmic trading. It has revolutionized the financial industry. It enables automated and high-frequency trading strategies based on real-time market conditions.
Operational Efficiency
Business analytics helps organizations streamline operations, enhance cost-saving measures, and improve overall efficiency. By analyzing internal data, companies can notice areas where inefficiencies exist and implement process improvements to optimize performance. Financial institutions use analytics to check workflow processes, distribute resources effectively, and enhance customer service experiences.
Automated dashboards and AI-driven analytics tools offer real-time insights to executives. These insights enable them to make data-driven decisions. Such decisions enhance productivity and profitability.
Additionally, predictive maintenance business analytics can help reduce operational downtime and prevent system failures in financial institutions.
Why Real-Time Data Analytics is Crucial for Financial Decision-Making
Financial markets are highly volatile, and decisions made in real-time can significantly impact a company’s bottom line. Here are some key reasons why real-time analytics is indispensable:
1. Enhanced Risk Assessment
With real-time data, financial institutions can assess risks instantly, making risk management more proactive than reactive. Banks and investment firms can monitor ongoing transactions. They also watch market shifts and geopolitical events. This monitoring helps detect potential risks. Then, they can take preventive measures.
For example, an unexpected economic downturn or political instability can trigger automated alerts. This allows businesses to adjust their financial strategies suitably. Companies can build dynamic risk models by leveraging AI and machine learning. These models continuously update and refine themselves. They use real-time data inputs. This ensures robust risk assessment mechanisms.
2. Improved Investment Decisions
Investors and financial analysts heavily rely on real-time analytics to track market trends, news, and asset fluctuations. Advanced trading platforms process vast amounts of live data from stock exchanges. These platforms enable traders to make split-second decisions. Such decisions maximize returns. Algorithmic trading uses AI-powered models to execute trades.
It thrives on real-time analytics. It does so by analyzing market trends, volatility, and liquidity in seconds. By having instant access to stock market insights, investors can make well-informed decisions. This reduces the likelihood of financial losses. It also increases profitability.
3. Better Customer Insights
Businesses analyze customer behavior in real-time to personalize financial services and enhance customer satisfaction. With real-time analytics, financial institutions can track customer spending patterns, credit behavior, and banking habits to tailor personalized solutions. Banks can proactively offer suitable credit options.
They can also propose investment plans or insurance policies based on a customer’s financial profile and historical transactions. Additionally, financial institutions can use chatbots powered by AI-driven analytics to give instant, data-backed recommendations. This ensures seamless customer experiences. It also improves client engagement.
4. Optimized Business Operations
By utilizing real-time financial data, companies can streamline operations, reduce costs, and enhance efficiency. Automated dashboards powered by business intelligence tools give executives with up-to-the-minute financial insights, allowing them to make well-informed strategic decisions.
Companies can track cash flow, revenue trends, and expenses in real-time, preventing financial bottlenecks and improving liquidity management. Real-time analytics helps in inventory management. It aids budgeting and forecasting by continuously analyzing expenditure patterns. This ensures optimal resource allocation and cost efficiency.
Tools & Technologies Driving Real-Time Financial Analytics
The adoption of cutting-edge tools and technologies has facilitated the growth of real-time analytics in finance. Some of the most widely used technologies include:
Artificial Intelligence (AI) & Machine Learning (ML)
AI and ML lead in financial analytics. They give powerful predictive modeling capabilities. This enables businesses to make highly correct forecasts. AI-driven algorithms can analyze massive datasets, detect complex patterns, and automate decision-making processes.
For instance, machine learning models can be trained to predict stock market fluctuations. They can also assess credit risks. Additionally, models improve loan approvals based on real-time behavioral analysis. Additionally, AI-powered chatbots and virtual assistants enhance customer support by providing personalized financial insights and recommendations.
Big Data Analytics
The financial sector generates vast amounts of structured and unstructured data daily, from customer transactions to global economic reports. Big data analytics allows financial institutions to process and analyze this information efficiently, deriving meaningful patterns and trends.
By leveraging big data, banks and investment firms can gain deeper insights into customer behavior, market conditions, and economic indicators. These insights drive more informed decision-making, from risk assessment to personalized financial services.
Moreover, big data analytics enhances fraud detection by identifying unusual transaction patterns that indicate fraudulent activity.
Cloud Computing
Cloud computing has revolutionized the way financial institutions store, access, and analyze real-time data. With cloud-based solutions, companies can scale their data infrastructure, ensuring seamless access to large datasets from anywhere in the world. Cloud platforms allow businesses to integrate various data sources, ensuring that real-time analytics tools operate efficiently and provide up-to-the-minute insights.
Moreover, cloud computing reduces operational costs. It eliminates the need for expensive on-premise servers and IT maintenance. This makes data analytics more accessible and cost-effective for financial firms of all sizes.
Blockchain Technology
Blockchain technology is increasingly being adopted to enhance transparency, security, and trust in financial transactions. Blockchain uses a decentralized ledger system. This system ensures that financial records are tamper-proof.
Therefore, it reduces the risk of fraud and unauthorized modifications. Real-time analytics in blockchain technology allows institutions to track and verify transactions instantly, providing enhanced visibility into financial operations.
Additionally, blockchain-powered smart contracts enable automated and secure transactions. They reduce the need for intermediaries. These smart contracts speed up processes like payments, loan approvals, and asset transfers. The integration of blockchain with AI-driven analytics further strengthens risk assessment and compliance monitoring.
Future Trends in Real-Time Financial Analytics
As technology continues to evolve, real-time data analytics will play an even more significant role in finance. Emerging innovations are shaping the future of financial analytics, leading to enhanced efficiency, security, and personalization. Below are some anticipated trends:
AI-Powered Automated Trading
Artificial intelligence (AI) has already transformed trading, but the future holds even greater advancements in AI-driven automated trading systems. These systems use machine learning algorithms to analyze vast amounts of real-time financial data. They detect patterns and execute trades within milliseconds.
By minimizing human intervention, AI trading enhances accuracy, reduces risks, and optimizes investment strategies. As AI evolves further, we can expect more sophisticated trading models. These models will adapt dynamically to market fluctuations. This adaptation ensures higher profitability and lower losses for investors and financial institutions.
Blockchain for Real-Time Settlements
Blockchain technology is set to revolutionize financial transactions by facilitating real-time settlements with enhanced transparency and security. Traditional banking systems often involve multiple intermediaries, resulting in delays and increased transfer fees.
With blockchain, financial transactions can be recorded in a decentralized and immutable ledger, enabling instant verification and settlements.
Smart contracts further automate compliance and execution, reducing the reliance on manual processing. As more institutions adopt blockchain, financial markets will experience faster and more secure transactions, reducing fraud and enhancing operational efficiency.
Personalized Financial Services
Real-time data analytics is paving the way for hyper-personalized financial services tailored to individual customer needs. Banks and financial institutions analyze real-time insights from customer transactions. They examine spending habits and financial goals. With this information, they can offer customized recommendations.
They also give tailored investment portfolios and credit options. AI-powered financial advisors will give users with real-time suggestions based on market conditions, helping them make more informed financial decisions. The future will see a shift towards fully personalized banking experiences. Every product or service will be uniquely tailored to the user’s financial behavior and preferences.
Regulatory Compliance Automation
Financial regulations are constantly evolving, and ensuring compliance is a major challenge for financial institutions. Real-time analytics, together with AI-driven automation, will be crucial for regulatory compliance. These tools will continuously oversee transactions, detect anomalies, and generate instant compliance reports.
Automated compliance systems will be capable of identifying suspicious activities, flagging regulatory violations, and streamlining reporting processes. This will not only reduce the risk of non-compliance but also improve efficiency by eliminating the need for manual auditing. The integration of AI and blockchain in compliance automation will further strengthen financial security and regulatory adherence in real-time.
Pros and Cons
Pros
- Improved Decision-Making: Real-time analytics provides up-to-date insights, enabling businesses to make well-informed financial decisions quickly.
- Enhanced Risk Management: Financial institutions can proactively detect and mitigate risks before they escalate, reducing financial losses.
- Fraud Prevention: AI-driven analytics identifies fraudulent activities in real time, protecting businesses and customers from financial crimes.
- Increased Operational Efficiency: Automated data analysis streamlines financial processes, reducing errors and increasing overall productivity.
- Better Customer Experience: Personalized financial solutions enhance customer satisfaction and engagement, leading to stronger client relationships.
- Competitive Advantage: Businesses that leverage real-time insights can respond swiftly to market changes and gain an edge over competitors.
Cons
- High Implementation Costs: Deploying real-time analytics solutions requires significant investments in technology and skilled personnel.
- Data Security Risks: Handling vast amounts of real-time data increases the risk of cyberattacks and data breaches.
- Complex Integration: Integrating real-time analytics tools with existing financial systems can be challenging and time-consuming.
- Dependence on Technology: Financial institutions rely heavily on automated analytics, which lead to vulnerabilities if system failures occur.
- Data Overload: Processing excessive data in real time can sometimes lead to information overload. This makes it difficult to extract meaningful insights.
- Regulatory Compliance Challenges: Real-time data processing must adhere to evolving financial regulations, requiring constant updates and monitoring
Conclusion
Real-time data analytics is revolutionizing financial decision-making by providing businesses with instant, data-driven insights. As financial markets become more complex and competitive, leveraging analytics will be crucial for maintaining a competitive edge. Companies that embrace real-time analytics will be better positioned to mitigate risks, improve investments, and enhance customer experiences. Investing in the right analytical tools and strategies today will shape the future of finance tomorrow. Want to stay ahead in financial analytics? Start leveraging real-time data analytics today!
FAQ: Business Analytics – The Increasing Importance of Real-Time Data Analytics in Financial Decision-Making
What is business analytics?
Business analytics refers to the use of data analysis tools and techniques to help organizations make better, data-driven decisions. It involves collecting large amounts of data. The process includes analyzing and interpreting this data to identify trends, patterns, and insights. These findings guide business strategies and actions.
What is real-time data analytics in business?
Real-time data analytics is the process of continuously analyzing data as it is created or received. This enables businesses to make immediate, informed decisions. This type of analysis enables organizations to act on data quickly. They can respond to changing conditions in real time. It prevents them from relying on outdated or delayed information.
How does real-time data analytics profit financial decision-making?
Real-time data analytics provides financial decision-makers with up-to-the-minute insights into market conditions, cash flows, investments, and other financial variables. This timely access to data helps businesses:
Make more informed, agile financial decisions
Notice and mitigate risks quickly
Optimize cash management and resource allocation
Improve forecasting accuracy and responsiveness
React faster to market changes, reducing delays in critical decisions
Why is real-time data analytics increasingly important in today’s financial landscape?
The financial landscape is more volatile and competitive than ever before. Real-time data analytics allows businesses to:
Keep pace with rapidly changing market trends and consumer behavior
Ensure that financial strategies are based on the most current information
Improve operational efficiency by quickly addressing inefficiencies
Gain a competitive edge by making quicker, smarter decisions in response to emerging opportunities or threats
How does real-time data analytics improve forecasting in finance?
By leveraging real-time data, companies can continuously update their financial models and predictions based on the latest trends. This makes financial forecasts more correct. They become reflective of the current market conditions. This leads to better budget planning, resource allocation, and strategic initiatives.
Can real-time data analytics help with risk management?
Real-time analytics allows companies to notice potential financial risks promptly. These include changes in market conditions, credit risks, and fraud activities. With timely insights, businesses can implement corrective measures quickly, minimizing the impact of risks on their bottom line.
What tools are used for real-time data analytics in business?
Several advanced tools and technologies are used to analyze real-time data, including:
Business Intelligence (BI) tools like Tableau, Power BI, and Qlik
Cloud-based platforms like Google BigQuery, Amazon Redshift, and Microsoft Azure
Data visualization tools for quick and effective interpretation of real-time data
Machine learning algorithms for predictive analytics and anomaly detection
Enterprise Resource Planning (ERP) systems that integrate real-time financial data across the organization
How does real-time data analytics support decision-making in stock market trading or investments?
In stock market trading, real-time data analytics helps investors track market fluctuations, price movements, and other financial indicators instantly. This allows traders to make more informed decisions, potentially improving investment returns and reducing losses. Algorithms and AI-driven insights can also automate trades based on real-time data, optimizing portfolio performance.
What challenges do businesses face when implementing real-time data analytics?
Some challenges include:
Data quality and consistency: Ensuring that real-time data is correct and reliable
Integration: Combining real-time analytics with existing systems and workflows
Scalability: Managing large volumes of real-time data without affecting performance
Data security and privacy: Ensuring that sensitive financial data is protected during real-time analysis
Skilled personnel: Finding qualified staff to analyze and interpret real-time data effectively
How can businesses start adopting real-time data analytics in their financial operations?
Businesses can take the below steps to implement real-time data analytics in financial decision-making:
Invest in the right tools and platforms that support real-time data processing and analysis
Train staff in data analytics and decision-making based on real-time insights
Integrate real-time data sources into existing financial systems for seamless data flow
Prioritize data governance to ensure accuracy, security, and compliance with regulations
Establish clear objectives for using real-time analytics in financial decision-making, improving cash flow management, optimizing investments, or enhancing risk mitigation
Is real-time data analytics only useful for large companies?
No, real-time data analytics is beneficial for businesses of all sizes. Large companies have more resources to invest in advanced analytics tools. However, smaller businesses can still leverage real-time data insights. They can use cloud-based platforms, cost-effective analytics tools, and specialized software designed for their needs.
What is the future of real-time data analytics in financial decision-making?
The future of real-time data analytics in finance looks promising as AI, machine learning, and automation continue to evolve. As more businesses adopt real-time analytics, the technology will become more accessible. This adoption allows for faster and more correct financial decisions. Additionally, advancements in predictive analytics will enable companies to expect trends and outcomes with greater precision.